Julian Straub
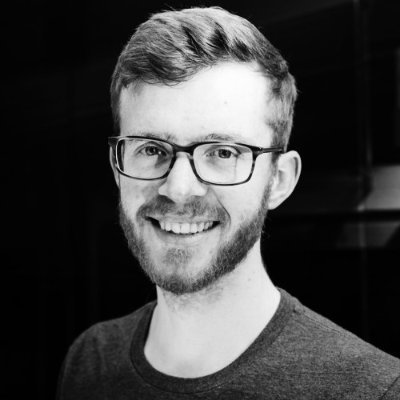
Julian Straub is a Lead Spatial AI Research Scientist at Meta Reality Labs Research (RLR) working on Computer Vision and 3D Perception. Before joining RLR, Julian obtained his PhD on Nonparametric Directional Perception from MIT, where he was advised by John W. Fisher III and John Leonard within the CS and AI Laboratory (CSAIL). On his way to MIT, Julian graduated from the Technische Universität München (TUM) and the Georgia Institute of Technology with a M.Sc. He did his Diploma thesis in Eckehard Steinbach’s group with the NavVis founding team and in particular with Sebastian Hilsenbeck. At Georgia Tech Julian had the pleasure to work with Frank Daellart’s group.
Email Resume CV Scholar Twitter Github
Research
My current research interests are problems that involve 3D localization, recognition and description of objects and surfaces from egocentric video streams in scalable and generalizable ways.
![]() | 2025-03-20
Sonata: Self-Supervised Learning of Reliable Point Representations Xiaoyang Wu, Daniel DeTone, Duncan Frost, Tianwei Shen, Chris Xie, Nan Yang, Jakob Engel, Richard Newcombe Hengshuang Zhao, Julian Straub, CVPR 2025 paper github weights Self-supervised learning of powerful and reliable point cloud representations. Sonata features improve linear probing accuracy by 3x on ScanNet and performance by 2x when given only 1% of training data. |
![]() | 2024-09-29
EFM3D: A Benchmark for Measuring Progress Towards 3D Egocentric Foundation Models Julian Straub, Daniel DeTone, Tianwei Shen, Nan Yang, Chris Sweeney, Richard Newcombe Arxiv paper github slides talk The EFM3D benchmark measures progress on egocentric 3D reconstruction and 3D object detection. This accelerates research on a novel class of egocentric foundation models rooted in 3D space. A new model, EVL, establishes the first baseline for the benchmark. |
![]() | 2024-03-26
EgoLifter: Open-world 3D Segmentation for Egocentric Perception Qiao Gu, Zhaoyang Lv, Duncan Frost, Simon Green, Julian Straub, Chris Sweeney We show how to reconstruct and instance segment egocentric Project Aria data using Gaussian Splats. |
![]() | 2023-10-27
Omni3D: A Large Benchmark and Model for 3D Object Detection in the Wild Garrick Brazil, Abhinav Kumar, Julian Straub, Nikhila Ravi, Justin Johnson, Georgia Gkioxari Omni3D repurposes and combines existing datasets resulting in 234k images annotated with more than 3 million instances and 98 categories. We propose a model, called Cube R-CNN, designed to generalize across camera and scene types with a unified approach. |
![]() | 2023-10-27
Orienternet: Visual localization in 2d public maps with neural matching Paul-Edouard Sarlin, Daniel DeTone, Tsun-Yi Yang, Armen Avetisyan, Julian Straub, Tomasz Malisiewicz, Samuel Rota Bulo, Richard Newcombe, Peter Kontschieder, Vasileios Balntas We introduce the first deep neural network that can accurately localize an image using the same 2D semantic maps that humans use to orient themselves. OrienterNet leverages free and global maps from OpenStreetMap and is thus more accessible and more efficient than existing approaches. |
![]() | 2023-10-27
Pixel-Aligned Recurrent Queries for Multi-View 3D Object Detection Yiming Xie, Huaizu Jiang, Georgia Gkioxari, Julian Straub CVPR 2023 paper supplementary code PARQ detects 3D oriented bounding boxes of objects from short posed video sequences. |
![]() | 2023-08-24
Project Aria: A New Tool For Egocentric Multi-Modal AI Research Jakob Engel, Kiran Somasundaram, Michael Goesele, …, Julian Straub, … Richard Newcombe The Project Aria device from my team at Meta Reality Labs Research is an egocentric, multi-modal data recording and streaming device with the goal to foster and accelerate research. Join Project Aria. |
![]() | 2022-06-18
Nerfels: Renderable Neural Codes for Improved Camera Pose Estimation Gil Avraham, Julian Straub, Tianwei Shen, Tsun-Yi Yang, Hugo Germain, Chris Sweeney, Vasileios Balntas, David Novotny, Daniel DeTone, Richard Newcombe Image Matching Workshop CVPR 2022 paper workshop We propose to represent a scene as a set of local Nerfs, which we call Nerfels. Nerfels can be used for wide baseline relocalization. |
![]() | 2021-10-11
ODAM: Object Detection, Association, and Mapping using Posed RGB Video Kejie Li, Daniel DeTone, Yu Fan Steven Chen, Minh Vo, Ian Reid, Hamid Rezatofighi, Chris Sweeney, Julian Straub, Richard Newcombe ODAM is trained to detect and track 3D oriented bounding boxes from posed video. We globally optimize a super-quadric-based 3D object representation of the scene. |
![]() | 2020-10-27
FroDO: From Detections to 3D Objects Martin Runz, Kejie Li, Meng Tang, Lingni Ma, Chen Kong, Tanner Schmidt, Ian Reid, Lourdes Agapito, Julian Straub, Steven Lovegrove Richard Newcombe CVPR 2020 paper We introduce FroDO, a method for accurate 3D reconstruction of object instances from RGB video that infers their location, pose and shape in a coarse-to-fine manner. |
![]() | 2020-08-23
Deep Local Shapes: Learning local sdf priors for detailed 3d reconstruction Rohan Chabra, Jan E. Lenssen, Eddy Ilg, Tanner Schmidt, Julian Straub, Steven Lovegrove Richard Newcombe ECCV 2020 paper Deep Local Shapes (DeepLS) is a deep shape representation that enables encoding and reconstruction of high-quality 3D shapes without prohibitive memory requirements. |
![]() | 2019-10-27
Habitat: A Platform for Embodied AI Research Manolis Savva, Abhishek Kadian, Oleksandr Maksymets, Yili Zhao, Erik Wijmans, Bhavana Jain, Julian Straub, Jia Liu, Vladlen Koltun, Jitendra Malik, Devi Parikh, Dhruv Batra We present Habitat, a platform for research in embodied artificial intelligence (AI). Habitat enables training embodied agents (virtual robots) in highly efficient photorealistic 3D simulation. |
![]() | 2019-10-27
StereoDRNet: Dilated Residual StereoNet Rohan Chabra, Julian Straub, Chris Sweeney, Richard Newcombe, Henry Fuchs CVPR 2019 paper StereoDRNet enables the estimation of metrically accurate depth maps enabling high-quality reconstruction by passive stereo video. |
![]() | 2019-07-06
DeepSDF: Learning Continuous Signed Distance Functions for Shape Representation Jeong Joon Park, Peter Florence, Julian Straub, Richard Newcombe, Steven Lovegrove We introduce neural shape representations in the form of a neural network that can be queried for signed distance values (SDFs). |
![]() | 2019-06-13
The Replica Dataset: A Digital Replica of Indoor Spaces Julian Straub, Thomas Whelan, Lingni Ma, …, Michael Goesele, Steven Lovegrove, Richard Newcombe The Replica dataset consists of 18 high resolution and high dynamic range (HDR) textured reconstructions with semantic class and instance segmentation as well as planar mirror and glass reflectors. |
![]() | 2018-08-01
Reconstructing scenes with mirror and glass surfaces Thomas Whelan, Michael Goesele, Steven Lovegrove Julian Straub, Simon Green, Richard Szeliski, Steven Butterfield, Shobhit Verma, Richard Newcombe SIGGRAPH 2018 paper We reconstruct mirrors in scenes by detecting a marker on the scanning device. This solves one of the most common failure cases of indoor reconstruction. |
![]() | 2017-09-18
Direction-Aware Semi-Dense SLAM Julian Straub, Randi Cabezas, John J. Leonard, John W. Fisher III Arxiv paper Toward fully integrated probabilistic geometric scene understanding, localization and mapping, we propose the first direction-aware, semi-dense SLAM system. |
![]() | 2017-05-15
Nonparametric Directional Perception Julian Straub PhD Thesis thesis From an indoor scene to large-scale urban environments, a large fraction of man-made surfaces can be described by only a few planes with even fewer different normal directions. This sparsity is evident in the surface normal distributions, which exhibit a small number of concentrated clusters. In this work, I draw a rigorous connection between surface normal distributions and 3D structure, and explore this connection in light of different environmental assumptions to further 3D Perception. |
![]() | 2017-02-01
The Manhattan Frame Model -– Manhattan World Inference in the Space of Surface Normals Julian Straub, Oren Freifeld, Guy Rosman, John J. Leonard, John W. Fisher III TPAMI paper We introduce the Manhattan Frame Model which describes the orthogonal pattern of the Manhattan World in the space of surface normals. |
![]() | 2017-01-01
Bayesian Inference with the von-Mises-Fisher Distribution in 3D Julian Straub All you want to know about Bayesian inference with von-Mises-Fisher distributions in 3D. |
![]() | 2016-03-15
Efficient Global Point Cloud Alignment using Bayesian Nonparametric Mixtures Julian Straub, Trevor Campbell, Jonathan P. How, John W. Fisher III CVPR 2017 paper Use branch-and-bound to globally align two point clouds using nonparametric distribution estimates of their point and normal distributions. |
![]() | 2015-12-07
Semantically-Aware Aerial Reconstruction from Multi-Modal Data Randi Cabezas, Julian Straub, John W. Fisher III ICCV 2015 paper We propose a probabilistic generative model for inferring semantically-informed aerial reconstructions from multi-modal data within a consistent mathematical framework. |
![]() | 2015-12-05
Streaming, distributed variational inference for Bayesian nonparametrics Trevor Campbell, Julian Straub, John W. Fisher III, Jonathan P. How NeurIPS 2015 paper How to perform Bayesian nonparametric inference on streaming data in a distributed parallelizable way. |
![]() | 2015-09-28
Real-time Manhattan World Rotation Estimation in 3D Julian Straub, Nishchal Bhandari, John J. Leonard, John W. Fisher III IROS 2015 paper We show how to use surface-normals to estimate the global drift-free rotation to a surrounding Manhattan World – a real-time “structure compass”. |
![]() | 2015-05-19
A Dirichlet Process Mixture Model for Spherical Data Julian Straub, Jason Chang, Oren Freifeld, John W. Fisher III We introduce a nonparametric Dirichlet Process mixture model over the sphere via tangent-space Gaussian distributions. This allows modeling complex distributions on the sphere. |
![]() | 2015-05-19
Small-Variance Nonparametric Clustering on the Hypersphere Julian Straub, Trevor Campbell, Jonathan P. How, John W. Fisher III CVPR 2015 paper We show how to derive a fast nonparametric DP-means algorithm, DP-vMF-means, for directional data like surface normals. This allows us to analyze surface normal distributions of depth images. |
![]() | 2014-10-27
A Mixture of Manhattan Frames: Beyond the Manhattan World Julian Straub, Guy Rosman, Oren Freifeld, John J. Leonard, John W. Fisher III CVPR 2014 paper We propose a novel probabilistic model that describes the world as a mixture of Manhattan Frames: each frame defines a different orthogonal coordinate system. |
![]() | 2013-09-15
Fast Relocalization for Visual Odometry using Binary Features Julian Straub, Sebastian Hilsenbeck, Georg Schroth, Robert Huitl, Andreas Möller, Eckehard Steinbach ICIP 2013 paper We use locality-sensitive hashing to speed up binary feature retrieval for fast camera relocalization. |
Writeups
Community Service
Reviewing CV:
- CVPR 2015/2018/2019/2020/2022/2023
- ICCV 2023
- ECCV 2020
- TPAMI 2016/2017
Reviewing ML:
- NeurIPS 2015/2022/2023
- ICLR 2023/2024
- AISTATS 2015
- ICML 2015
Reviewing Robotics:
- ICRA 2016/2023
- IROS 2016